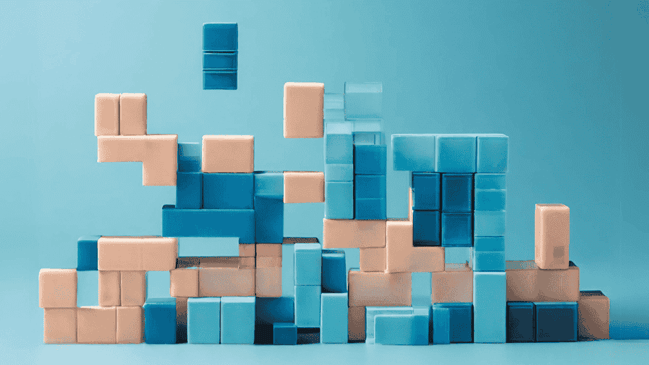
Trusted Data: The Foundation of Data Analytics and AI, and Effective Data Governance
- By:
- George T. Tziahanas |
- April 24, 2024 |
- minute read
The concept of trust seems simple enough; in essence, implying confidence or the ability to rely on something - in this case data. In reality, trust in data is more complicated, because of its enormous volume and velocity in modern enterprises arising from a myriad of applications and use of data to drive decisions and automate processes.
The widescale use of data analytics platforms, and increasingly AI-enabled solutions, is leading to a voracious appetite for data of all types. However, as I wrote in a previous blog, Wrong at the Speed of AI, the quality and appropriate use of data is critical to deriving value while managing data risk. Meanwhile, ¹Gartner Research estimates by 2025, 30% of GenAI projects will be abandoned after proof of concept, due to poor data quality, inadequate risk controls, escalating costs, or unclear business value. (Arun Chandrasekaran, Distinguished VP Analyst at Gartner Highlights from Gartner Data Analytics Summit 2024)
So what are the key attributes that drive trust in data? At Archive360, we have built core elements into our platform, to provide the basis of trust in the data we govern.
Archive360 Platform Core Elements:
1. Data Lineage and Provenance:
This includes maintaining a record of the source of the data, its origin, ownership, and any changes in metadata (if permitted) throughout its life cycle within our platform. Uniquely, it also means maintaining rich metadata and all the underlying documents or artifacts from which it is derived. This provides necessary transparency to consumers of the data, especially across large volumes and timeframes.
2. Data Authenticity:
Maintaining a clear chain of custody for all data within our platform, storing objects in their native forms, and hashing objects received to demonstrate data remains unchanged is key. In addition, we maintain a full audit history for each object, and for all actions with respect to changes in policies and controls. This means analytics, legal and compliance, records, and business teams are certain the data remains in its original form.
3. Data Security:
Archive360 deploys each customer in their own, dedicated cloud-tenant, tailoring security controls as needed based on their requirements. This as opposed to traditional SaaS platforms that require customers to accept the security protocols of the vendor. The platform provides options for multiple levels of data encryption, including field level encryption and masking. Customers can also maintain their own encryption keys, separate from the Archive360 environment for additional levels of data security. This allows organizations greater control and flexibility in meeting their security requirements.
4. Data Entitlements:
Controlling access and use of data is critical to managing risk, while delivering the right data for analytics and AI solutions. Within Archive360, enterprises can entitle data in as granular a manner as needed, including at an object or field level, based on user or system profiles. In addition, Archive360 can leverage an organization’s entitlement engine, to provision and verify access rights to data. This means the right data is available to users and systems who are entitled to access it, while restricting or limiting access to those who are not.
5. Data Compliance:
Many organizations are subject to regulatory and statutory retention obligations, which include prescriptive requirements around data immutability, resiliency, and sovereignty. In addition, data privacy needs are extensive for many enterprises. The Archive360 platform incorporates broad-based policy capabilities, which establish appropriate compliance controls across all types of data. With ever-increasing data compliance requirements, this capability is critical to organizations.
6. Data Classification:
Establishing the nature of a set or type of data is important since it establishes the governance requirements. Our unique, class-based data management architecture allows organizations to govern structured data, semi-structured data, and structured sets of data in one single platform. Each class can have a unique schema, which allows organizations to manage such diverse sets of data without a one-size-fits-all fixed ontology. This makes publishing data to analytics and AI solutions more effective, versus the data being unnecessarily manipulated to force it into an inflexible data structure.
7. Data Normalization:
Enterprises work with a range of systems that create, move, and process data. Establishing common definitions and formats of metadata is important for governance requirements, but also downstream use in analytics and AI solutions. Clearly defined schemas is an important element, along with tools that can transform or map data to maintain consistent, normalized views of related data. The platform can also leverage existing metadata management or organizational taxonomies in building Archive360’s ingestion pipelines.
Much of the data that customers govern in their Archive360 platform has compliance or long-term retention requirements or may have been moved into our platform as part of decommissioning aging applications. These sets of data were traditionally unavailable to AI and analytics tools, in part because of difficulty consuming the data, and in part because of the strict data compliance requirements outlined above.
A core differentiator of the Archive360 platform is not just our ability to govern all types of data across a range of obligations but in the publishing of trusted data to analytics and AI solutions. In addition, AI and analytics solutions are creating enormous volumes of data that will be increasingly subject to governance and compliance requirements. These data sets can be moved into a dedicated data governance platform like Archive360 as they age while remaining available if needed for those solutions. Trust is central to analytics and AI, along with effective governance; and that’s exactly what has been designed and built into Archive360’s platform. If this blog sparked your interest, talk to one of our experts today.
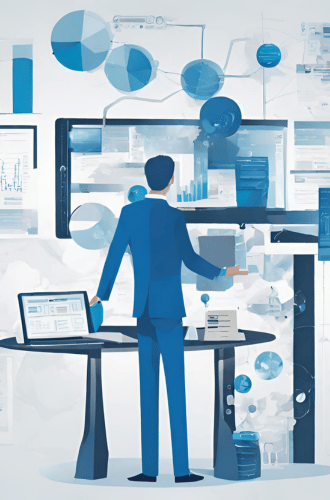
UPCOMING WEBINAR
Regulating the Robots: A Global Survey of AI Legislation and Regulation
Register now to learn:
- Review actions and commentary from regulators to date related to AI under existing authorities and examine emerging global statutory frameworks to govern AI.
- Explore potential legislation in various U.S. states and identify commonalities across these proposals.
- Outline suggested near-term roadmap items to prepare for what will most likely impact organizations.
George Tziahanas, AGC and VP of Compliance at Archive360 has extensive experience working with clients with complex compliance and data risk related challenges. He has worked with many large financial services firms to design and deploy petabyte scale complaint books and records systems, supervision and surveillance, and eDiscovery solutions. George also has significant depth developing strategies and roadmaps addressing compliance and data governance requirements. George has always worked with emerging and advancing technologies; introducing them to address real-world problems. He has worked extensively with AI/ML driven analytics across legal and regulatory use cases, and helps clients adopt these new solutions. George has worked across verticals, with a primary focus on highly regulated enterprises. George holds an M.S. in Molecular Systematics, and a J.D. from DePaul University. He is licensed to practice law in the State of Illinois, and the U.S. District Court for the Norther District of Illinois.