Description:
In this podcast episode titled "Navigating the AI Governance Landscape," George Tziahanas, VP of Compliance at Archive 360, engages in a compelling conversation with Michael Rasmussen, an esteemed analyst specializing in governance, risk, and compliance (GRC). Throughout the episode, listeners will gain insights into:- The pivotal role of data governance in the context of AI and analytics, shedding light on its significance as the cornerstone for AI model governance and risk management.
- The intricate regulatory framework surrounding AI deployments, including considerations such as legal liabilities and protection of sensitive information.
- The fundamental principles of data governance ensuring data quality, security, and compliance, indispensable for fostering effective AI and analytics ecosystems.
- Analysis of the EU AI Act, elucidating its mandates pertaining to data quality, protective measures, and ethical standards adherence.
- The importance of trust and governance in both data and AI systems, advocating for a holistic approach that enhances integrity and confidence in organizational data practices.
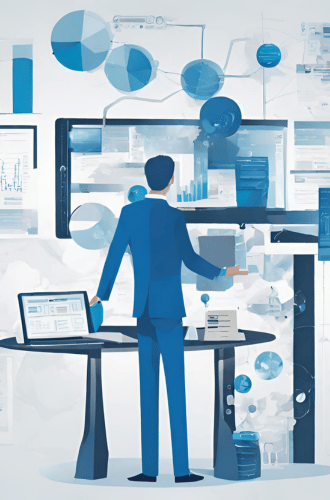
WHITEPAPER
Data GRC Management by Design
An Integrated Approach to Data Governance & Management for Today's Enterprise Organization. Read this whitepaper to learn:
- Data GRC Management by Design
- The Role of Data & Data Processes is Changing
- A Framework of Data GRC Processes
- The Data GRC Information & Technology Architecture
Speakers
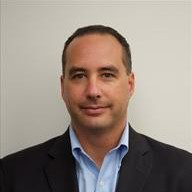
George Tziahanas
AGC and VP of Compliance
Archive360
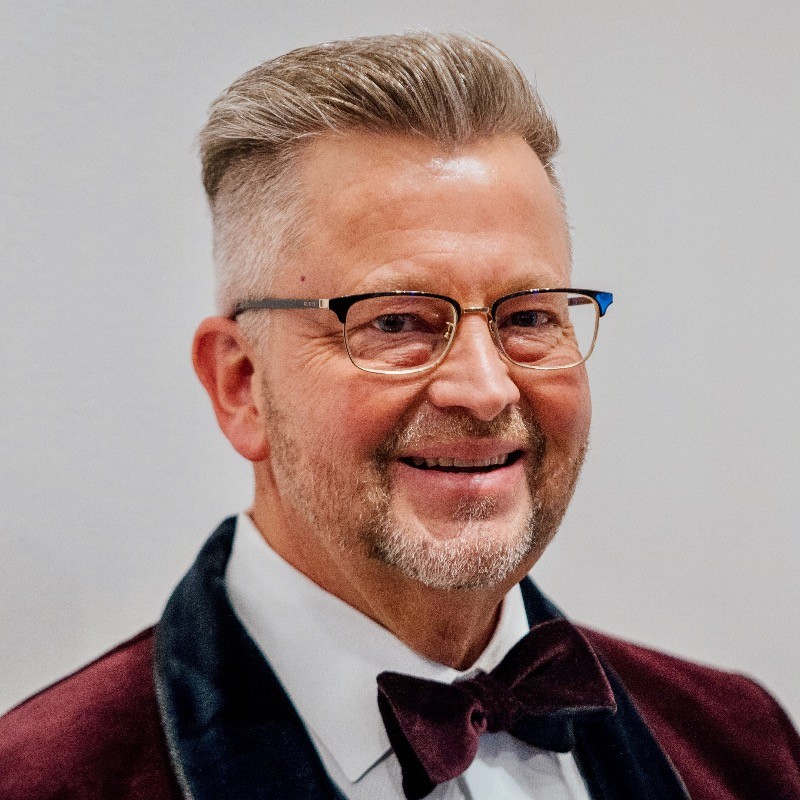
Michael Rasmussen
GOVERNANCE EXPERT
GRC 20/20
Transcript
George Tziahanas:
Welcome to this week's webcast for Archive 360, titled The Growing Importance of Data Governance in an AI-Driven World, Delivering on the Value of AI and Analytics While Managing the Risk. I'm George Tziahanas, the VP of compliance at Archive 360, and today we're fortunate to have pundit and analyst Michael Rasmussen, also known as the father of Terming GRC. First, thanks for joining us. I thought we might start with a conversation around, can we talk about value and risk. Everybody sees the value in ai. Everybody sees potential risk in ai, which we'll talk a bit more about, but a lot of talk also is data governance has got to be a part of this discussion as we move into this new AI and analytics-driven world. So where do you see data governance fitting into this broader concept?
Michael Rasmussen:
Well, from my perspective, data governance is the foundation for AI model governance and AI risk management. And we've got a lot coming at us with the EU AI Act, here in the states you have things like the NAIC, the National Association of Insurance Commissioners and their AI model audit rule that the 50 different states have to implement. That's some of the regulatory aspects, but the reality is it's sort of like the AI Wild West out there where organizations really don't even have good control over how AI is being used in their organizations.
And we've talked a lot about things like shadow IT. We now have shadow AI because anybody can go out to chat GPT and put information out there and process that information and rely on that information that could expose the organization to legal liability and a lot of other unwanted risks. Just was interacting on a micro simulation just last week in which the whole micro simulation and going through risk scenarios where an organization has a bunch of their data that was out there that was ingested into one of these learning models to be able to process something by some employee that wasn't authorized to use that.
And now all of a sudden that's publicly available because what you put in a chat GPT, guess what? It goes into the large language model that others can leverage and use as well. And so there's a lot of exposure. So to me, data governance is the foundation for good AI governance. You cannot really govern the artificial intelligence use in your organization without a solid foundation that's built on what I call data governance, risk management and compliance. If we have bad data going in, guess what? We're going to get bad results coming out of the AI model. But that's only one of the risks because we need to make sure that data going into those models and things, particularly public models, is appropriate and isn't exposing the organization to disclosures that it doesn't want to disclose.
And so data governance provides a framework for data quality, security, compliance, which are the critical foundation for effective artificial intelligence and analytics. Data governance to me provides that foundation of trust and integrity. It ensures that the data used within these AI systems is accurate, timely, and reliable for what's going in, so we can be more reliant on what comes out of the AI models. And of course, data governance fits in the whole regulatory compliance scheme. We'd have to adhere to laws like the EU AI Act, but other laws like GDPR and California CCPA and others that weren't directly written in any way because of AI, but definitely have AI and data governance implications.
George Tziahanas:
Yeah, we'll get to the regulatory piece here in a second. I wanted to kind of come back to this concept of trust, the analytics applications that are out there and platforms along with AI as it starts to emerge more broadly, trust in the data and trusted sources of data are really important. And you started to get to that, and we think of it in the context of providence and lineage and security classification, normalization of data scale. Just maybe talk about some of those items as well in this concept of trust.
Michael Rasmussen:
Yeah, trust is integral to the organization right now for ensuring that the organization of integrity, that it's being governed properly from the overall organization perspective, but down particularly in the data governance perspective, that we have good control over our data, the use of that data. And of course within AI as well, you mentioned the providence and lineage. I mean, it's critical that our organizations can track the source and history of data to ensure its integrity and the appropriate use of that data. And again, that's much broader than just AI, but it has specific implications on the data that goes into AI models. We should also strongly consider security and implementing robust security measures to protect data from unauthorized access and breaches because maybe we have had some type of confidence in that data and the data input that goes into the AI models.
But if somebody could compromise that data and all of a sudden the organization's making decisions through AI based on corrupted data because somebody has tainted that data, that's a big issue. We also need to have clear and strong classification normalization where we can categorize data accurately and ensure it is in a consistent format that can be used effectively by AI systems and know when, how, where, why, and who is using that data in those AI systems. And that all helps us with trust. And of course, we should also look at scale. We need to be able to ensure that data systems are scalable and can handle the increased data loads that comes from advanced analytics with artificial intelligence.
George Tziahanas:
Excellent. Scale I think we'll come back to when we get into the regulatory piece here in a second, which is the scale of actually starting to manage the things that will be subject to reporting and subject to record keeping, and then obviously production potentially as well. So let's switch a little bit to the regulatory landscape. And a lot's been written around the EU AI act, which we can talk about in a minute, but I'm kind of more interested to talk a little bit about a joint letter that was published by a number of the regulators here in both the Consumer Finance Protection Bureau, along with the DOJ and some other regulators. And then recently the SEC in late 2023 issued a record request to RIAs on their use of AI and a broad request around how they use AI, the models, the data, and all those things as well. So I guess a long way of asking, is it already regulated?
Michael Rasmussen:
Well, in one respect, particularly in the United States, we have regulation through our love for lawsuits. We're the number one litigious country in the world. There's a lot of organizations that are looking at AI governance because of the increased exposure to legal liability and lawsuits, and particularly things like class action lawsuits from the inappropriate use of AI, particularly around personal information and things like that. And so that's one aspect of it.
But you do bring up these other examples like the joint letter and the SEC record request with the RIAs, right there, what you're seeing is that there's existing laws and things that are on the books that can already be extended for AI, but then there's AI specific laws like we'll talk about in a minute, like the EU AI Act. But existing laws, particularly around data governance and things like that do apply to AI, they just have to be interpreted in the AI context and use. But also in the joint letter example and things, I think what we're also seeing is that the regulators out there are flexing their muscles and being able to gather information and show that this is coming. And so where some jurisdictions, like the European Union have very, very broad legislation that's enacted now, others, the regulators are taking existing things on the books, but also showing that they're looking at this more deeply. And there's more to come.
George Tziahanas:
The EU AI Act obviously is getting a lot of press. Maybe let's break down a little bit about what is it actually under the covers, what does it actually require and how it mirrors, by the way, a number of pieces of legislation at the state level here developing in the US?
Michael Rasmussen:
Yeah, so the EU AI Act is very broad and has a global impact. I've already had conversations around the world that they feel that they've got to address the EU AI Act because they have operations in Europe or they have significant processes and data on EU citizens in that context. And so the EU AI Act has a very broad scope. A lot of EU regulation does, whether it's EU CSRD or EU GDPR and so many more, the EU writes regulation so it really does have a global impact, and that's one thing to consider. The other consideration is how they approach fines. With violations of the EU AI Act, they can go after up to 7% of your global turnover in revenues globally, not just in the EU, but globally. That's significant potentials for fines on organizations. And so organizations around the world are addressing the EU AI Act because it is the broadest regulation that we have out there right now.
It does take a risk-based approach where there's high-risk systems that you need to keep in mind to address this. That's the central piece to it. And then there's more moderate-risk systems that come into play that you have to have some type of risk and control around, but not as much detailed. But then there's a lot of AI use out there that is a very low-risk, and so that's not as big of a concern out there. When we look at this AI Wild West, I recently wrote a blog that there's a new law in town and that Wild West is getting tamed a little bit right now. The EU AI Act categorizes AI systems based on the level of risk. Again, as they pose high-risk systems, particularly, the high-risk scenarios, organizations must ensure the data quality, which really gets into data governance that we're talking about, enhanced protection measures, and adherence to ethical standards.
The EU AI Act also bans specific uses of AI that are considered harmful, such as certain types of biometric identification and social scoring systems. But the AI systems that are classified as high-risk encompass technologies that are used in various critical sectors like critical infrastructure such as transportation systems where AI can significantly impact citizens safety and health, education and vocational training where AI scores exams, product safety components where AI applications might be used, like in robot-assisted surgery and medical devices, employment and worker management, which has a broad impact such as a CV sorting software for recruitment, which can affect employment and self-employment opportunities, and then might have issues impacting diversity and inclusivity as well as harassment and discrimination on the other side of it. You have essential services. So the examples there for AI can be in credit scoring that could deny loans to individuals, law enforcement, migration, asylum and border control, justice and democratic processes, all those are critical.
So organizations operating high-risk AI systems under the EU AI Act need to have a thorough AI risk assessment and mitigation strategies. Right there foundationally, you're going to have to have an AI inventory, but that also includes your data inventory and governance as well that goes into those models. You need to provide assurance of high-quality data sets, again, data governance, to minimize risk and avoid biased outcomes. You should have comprehensive, not should, but you need to have comprehensive activity logs for result traceability and AI usage, in-depth documentation for how AI is used and how it was validated, clear, detailed information on AI models to be used by AI users and so forth. So I mean, there's a lot to the EU AI Act that requires validation and control of not only the models, but the data governance aspect of it as well.
George Tziahanas:
Yeah, no, absolutely. And so what's interesting too is if you go back and you look at the, bring this back a little bit first, I think we all agree that this is still very much managing the risk to achieve the value, because I think everybody realizes the value of AI and analytics is really untold at some level. We will not even realize what it looks like a few years from now. But what's interesting is joint letterhead kind of broken things down and potential sources of challenges or problems around the data and the data sets, the models, and then how that system is designed and used. And then as you look at the EU Act, if you look at how the regulators are looking at things today and some of the state actions, we kind of keep coming back to those same themes. And it's what is the data? Do I understand the data? Do I have governance around the data? Do I have the right record keeping around it? And then are these systems designed and tested to be accurate, robust, and secure? And those elements are used throughout all of this legislation.
Michael Rasmussen:
In the financial services space. We've had model risk management for a very long time, and in financial services, we're seeing that a lot of those processes for what we call model risk management are being adapted and extended to AI models.
George Tziahanas:
Absolutely. It's actually one of the forms of data some of our financial services clients use us to leverage for their governance of those models, especially over the long term, the models and the data that goes around them, a tremendous amount of data, significant, and so we provide that platform for them. But to your point, this is just another model in many instances, you can call it an AI model versus a Monte Carlo model. What's really the difference, right?
Michael Rasmussen:
The difference being the model itself and how it leverages AI, but they're all models.
George Tziahanas:
Exactly. Yeah. And maybe that serves as an interesting, to your point, analog that people should look at to the extent that they do have risk management already in place or things like that, this is a place that they can look to extend for analytics and for AI as well. What should organizations be doing today? Obviously analytics platforms are broadly deployed. AI platforms are deployed at various levels, approved and unapproved, to the extent that that happens, where do organizations start today to get the value but still manage the risk?
Michael Rasmussen:
I think you bring up an interesting point there because good risk management, if we go by the definition of risk in ISO 31,000, the international standard on risk management, is risk is the effect of uncertainty on objectives. And if we're going to really approach strong risk management with AI, we need to clearly understand what is the objectives and purpose of AI in each use case for AI in the organization, and then what's that risk and uncertainty that we face in using that AI and how do we mitigate that? But then also, how do we act with integrity of it? When we talk about, I interacted a lot with Archive 360 on what I call data GRC, data governance, risk and compliance. The same thing happens with artificial intelligence. We have AI governance, risk and compliance where we want to reliably achieve the objectives in our use of AI, but manage the uncertainty and risk as we use AI and act with integrity to address the ethical, legal and regulatory concerns in our use of AI as well.
All those are absolutely critical and require strong data governance for the data coming into the AI model, so that AI input as well as the model processing component and the output component. But there's a lot of places where artificial intelligence can fail, such as the dynamic and changing environment. Our AI models might be built for a very specific purpose at a very specific time. And as the business and the economic and broader environments change, that AI model, if it doesn't change and adapt with it, could be old and outdated and giving bad information to the organization, which also includes the data itself that's changing that goes into that. You have the lack of governance and control of AI in the organization, sort of the wild west because anybody can go out to Chat GPT and start using it and other sources of AI. But the critical thing is to understand that artificial intelligence is more than the model processing component in the AI itself.
It also involves that data input of the data coming in, which requires strong data governance and errors in that data that's input into the models mean that we're going to get garbage in garbage out. And so we need good strong data governance and control, not just for the quality of the data itself, but also as we've stated already, the security of that data. I mean, there's so much I can go into just on and on and on here, but organizations in response need to have an integrated approach to AI governance and data governance, and the oversight of all this, being able to manage the lifecycle of data and the lifecycle of AI in the organization and be able to address this strategically with technology to be able to manage the data and govern the data properly in the organization as well as the AI models themselves.
George Tziahanas:
Well, maybe that's a good place to look at closing this out. Maybe we return back to that concept of trust and governance. So you have to have the ability to have trusted sets of data that includes all the things we talked about before, the security, the provenance, classification scale, and then just good governance practices with your data is really how you derive the value out of analytics and AI without going too far on the risk spectrum.
Michael Rasmussen:
Most definitely. And in the words of our favorite fictional Premier League coach and philosopher, Ted Lasso, doing the right thing is never the wrong thing. In context here. Yeah, there's laws and regulations, but at the end of the day, AI is critically important to the organization to be able to leverage for value to the organization, but we need to govern it properly. To me, it's not just about compliance with the laws and regulations, but it's approaching data governance and AI governance in a way to ensure that we're doing the right thing for the organization and the communities it serves, whether it's employees or clients or the broader community, that there's proper governance of data and AI use out there. Doing the right thing is never the wrong thing. And so at the core of this, to me, organizations shouldn't approach this like, "How do I meet the letter of the law?" But, "How do we address data governance and AI governance in a way that enhances the organization's integrity and trust factor?"
George Tziahanas:
All right, well, great, Michael, as always, it's been a pleasure talking to you and really appreciate your time and I look forward to chatting again.
Thank you!
Thank you for tuning in today to the Data Governance 360 podcast presented by Archive 360, trusted by enterprises and government agencies around the world to support them at all stages of their data governance journey. To subscribe to our series or to learn how you can navigate the intricate challenges of data management and governance in today's digital landscape, visit archive360.com/podcasts.
Questions?
Have a question for one of our speakers? Post it here.